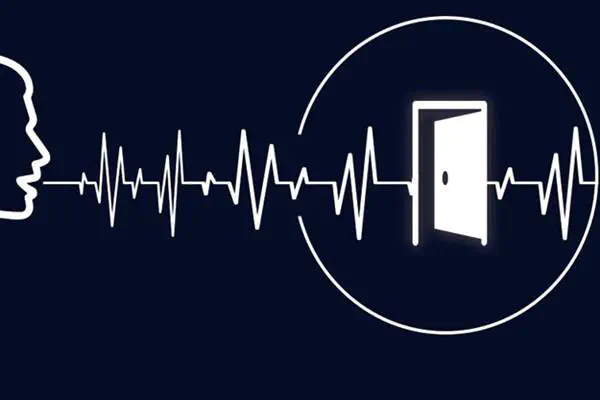
This project is related to my doctoral topic including speaker recognition, antispoofing, and robustness.
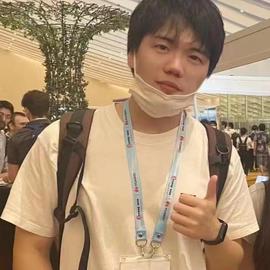
Chang ZENG 曾畅 曾 暢 (ソウ チョウ)
Ph.D. Candidate
My research interests include speech signal processing, speaker recognition, antispoofing, and singing voice synthesis.
Publications
Audio Deep Synthesis Detection, Spectro-temporal, Domain Adaptation, Self-Supervised Learning, Frame transition, Greedy Fusion.
Xiaohui Liu,
Meng Liu,
Lin Zhang,
Linjuan Zhang,
Chang Zeng,
Kai Li,
Nan Li,
Kong Aik Lee,
Longbiao Wang,
Jianwu Dang
We propose a fake audio detection (FAD) system using speaker anonymization (SA) to enhance the distinction between synthetic and natural speech. The key strategy is to remove speaker-specific information from acoustic speech before it’s processed by a deep neural network (DNN)-based FAD system. We employed the McAdams-coefficient-based SA algorithm to ensure that speaker information doesn’t influence DNN learning. Our system, based on a light convolutional neural network bidirectional long short-term memory (LCNN-BLSTM), was tested on the Audio Deep Synthesis Detection Challenge (ADD2022) datasets. Results indicate a 17.66% performance improvement in the first track of ADD2022 by eliminating speaker information from the acoustic speech.
Kai Li,
Sheng Li,
Xugang Lu,
Masato Akagi,
Meng Liu,
Lin Zhang,
Chang Zeng,
Longbiao Wang,
Jianwu Dang,
Masashi Unoki
Probabilistic linear discriminant analysis (PLDA) or cosine similarity has been widely used in traditional speaker verification systems as a back-end technique to measure pairwise similarities. To make better use of multiple enrollment utterances, we propose a novel attention back-end model that is applied to the utterance-level features. Specifically, we use scaled-dot self-attention and feed-forward self-attention networks as architectures that learn the intra-relationships of enrollment utterances.
Chang Zeng,
Xin Wang,
Erica Cooper,
Xiaoxiao Miao,
Junichi Yamagishi
speaker recognition, audio-visual, lip biometrics, deep learning, visual speech.
Meng Liu,
Longbiao Wang,
Kong Aik Lee,
Hanyi Zhang,
Chang Zeng,
Jianwu Dang